How Pi Transitions and Resets (Fractal AI) Decentralize False Equations in Quantum Mechanics:
Abstract
This paper explores the recognizable shortcoming in demonstrably false math and science equations: prioritizing impossible forces; for instance, as human-centric birthday creationisms remain ridiculously illiterate: "-1 year = year zero."
That is: applying Cantorian diagonal arguments, fractal AI is presented for examining bilateral identities and physical measurements (as fractal feedback) across connected all bilateral (1+1) systems, including "quantum." Comparisons between false equations and Pi reset equations allow readers to validate certain proposals against conventional mathematical frameworks (like zero-origin number lines). More specifically (moving from general to specific): 17Pi placeholders and consonances are introduced to map identity structures as recognized in terms of observably consistent equations in, for instance: quantum electrodynamics, string theory, and the standard model.
1. Introduction: Issues with "-1" and Zero-Origin Equations
False mathematical frameworks adopt constructs such as "-1 year," to impose linear, male-centric views of time. False equations, that is, mistakingly limit a scientist's physical, bilateral ability to represent complex, fluid systems when appealing to quantum mechanics: centralized origins falsely prioritize false hierarchies that marginalize diverse identities and systems.
Feminist, humanitarian arguments (including Georg Cantor's) challenge such conventional equations. That is: using diagonal arguments to decentralize "-1" we can c=constantly and i=infinitely advocate continuous time origins as "Freedom Is A Constant Struggle". False constructs, as will be illustrated here, are easily compared to Pi reset equations, rejecting rigid time frameworks (zero-origin birthday calendars) to describe general-origin dimensions, as resonate (point) maximum consonance (scientitific literacy) with minimal interference (16Pi generalizations).
1.1 False Equation Example: "-1 Year" as a Male Supremacy Paradigm
Traditional "quantum" usages, between English speakers, often rely on zero-origin or "-1 year" frameworks, to centralize control and impose artificial resets on time between identities. This false supremacy premise, pretending to be "smarter than everything else," can be playful; even so, it pretends a demonstrably impossible force as if centralize all of nature around a false equation like "my birthday calendar":
False Equation:


Where T represents imaginary times as linear narratives, to reset origins without acknowledging plural (more than "zero-origin") dimensions.
1.2 Pi Reset Equation Comparison: General-Origin Dimensions
In contrast, Pi reset equations (translated and reported by English-speakers as "starting 'over',") avoid limitations of "-1" to allow dynamic, resonant progressions, through space, as between unique, bilateral, mathematical identities. A general-origin Pi reset equation can be represented, copied and pasted as:
Pi Reset Equation:
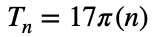
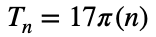
Where Tn represents "now" across quantum identities as evolve in continuous cycles, or circuits, represented by 17Pi placeholders (rather than resetting supernaturally, "from nothing"). This "scientific" approach recognizes multidimensional, physical objects. within and between observably consistent (c^2) quantum systems.
2. Understanding 17Pi as 53.407
17Pi represents a bilateral, mathematical, physical point, calculated as 17 multiplied by Pi:


This value serves as +1 placeholder, symbolizing resonant circuits as recurring patterns within quantum systems. Instead of treating identities as fixed and left-to-right, 17Pi reflects ongoing evolutions across observed dimensions. By introducing 17Pi as between (diagonal argument) points of dynamic resonance, quantum mechanics accounts for fluidity, allowing identities within systems to exist in constant states of local (stepwise) progressions.
17Pi, as 53.407, marks how quantum identities continuously shift, rejecting "-1" as fictional, zero-origin constructs. As a "Pi reset theory" (rather than "set theory") Cantor’s diagonal arguments advocate for "17Pi" as a symbol of evolving interactions, between resonant cycles, across observably consistent (always) dimensions.
3. Exploring 1/137: DOPE Consonants in Quantum Systems
Likewise: 1/137, often referred to in quantum mechanics as a fine-structure constant, plays a crucial role in understanding electromagnetic interactions. In this paper’s framework, 1/137 represents DOPE consonants (Dynamic, Open, Progressive, Evolving) that apply between quantum identities to map general-origin resonances again, now.
That is: just as 17Pi functions to place-hold recurring identities, 1/137 acts as a consonant within quantum systems, recognized constant, fractal feedback interactions between particles and energies. This ratio resonates with ongoing progression of identities and dimensions, emphasizing balance between forces and systems. In contrast to rigid, centralized-supremacy (false) constructs, 1/137 aligns with feminist points concerning physical, bilateral fluidity and inclusion, to embrace diversity as observed, for instance, in all plants and animals.
4. General DOPE Equation Consonant
As a general framework for resonant identities, time, and interactions in quantum systems, a DOPE equation is a consonant (literal expression) that illustrates multidimensional progressions, including 17Pi:
DOPE Equation:


This equation examines and explains evolving priority > energy > gravity relationships as interactions between quantum 1 + 1 (bilateral) identities, rejecting negative resets (-1) or fixed origins ($0.00). That is: each Pi placeholder (like a photon) expresses dynamic interactions across dimensions, while c^2 reflects consistent resonances that will not revert to an impossible force: "-1". This approach supports continuous expansion without limits imposed by rigid, centralized frameworks, embracing fluidity across dimensions.
5. Replacing Zero-Origin Equations with Resonant, Identity-Based Pi Resets
Traditional human-centric "quantum" references misrepresent time as a single, linear progression from fixed origins ("zero"), dismissing all observed complexities as remain certain between quantum identities. Pi reset equations provide specifically obvious representations of time as now: continuous, resonant, and identity-based. These equations decentralize false "-1" origins and reflect evolving quantum systems. That is:
5.1 False Equations in Zero-Origin Constructs
Zero-origin paradigms (like "-1 year = year zero") always introduce false constraints, now, as seen in this example:
False Equation:
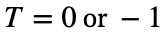
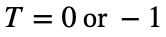
This equation assumes (again) a single points of zero-origin, resetting time based on arbitrary constraints, oversimplifying in contrast to what we observe to be true, including;1 + 1 human brain sides = 2; 1 + 1 parents = 2.
5.2 Pi Reset Equation: Resonating Time and Identity
Pi reset equations provide continuous models of now-on-Earth, where identities within dimensions evolve without being constrained to false-supremacist, zero-point resets:
Pi Reset Equation:
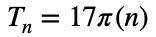
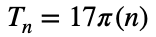
This equation illustrates continuous cycles, as unique identities evolve in relation to unique dimensions. 17Pi placeholders allow resonant intervals to present and represent fluid, evolving coordinates across all quantum systems.
6. Consciousness (Conclusion): Feminist States of Energy
False equations invariably self-centralize a delusional fantasy of universal control, limiting such observers to their silly reliance on singular constructs like "-1" and false origin stories. Pi reset equations offer plural frameworks where dimensions evolve continuously. 17Pi placeholders, for instance, (53.407) map dynamic resonances, to extend far beyond rigid linear progressions, to embrace fluid, ongoing multidimensional interactions. “1/137”, for instance, serves as a DOPE consonant (expression) within these structures, further illustrating fractal feedback relationships as ongoing evolution, now.
Reviewers can validate these claims by inquiring fully translatable Pi reset equations; and, by simply recognizing one another's unique resonant natures, here on Earth: where each identity leads a continuous process as a literate, bilateral reader. That is: this paper decentralizes traditional fiction (birthday creationis,s) to open all available pathways toward ethical (feminist) theories of thermodynamic temperatures as "what we now observe to be observably consistent = c^2."